Noisy Data
In general, most environmental data has a considerable amount of random noise it. The origin of this random noise is
- the phenomena itself has a lot of intrinsic variability - this is especially true with climate data
- the measurements are often difficult and imprecise
The example below shows a time series where the intrinsic variability completely swamps out any potential trend. To identify possible trends it is necessary to smooth the data.
A variety of techniques exist for this.
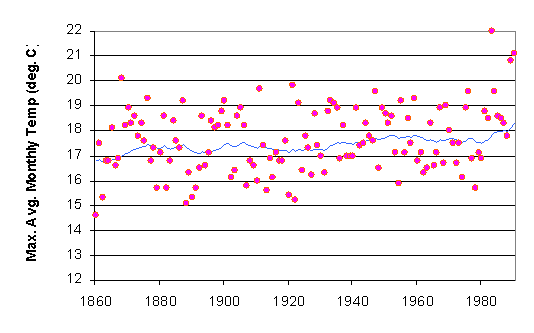
(blue line = smoothed trend)
Any data that has significant flucutations as a function of time may dominate the underlying wave form (trend with time). You therefore want to dampen out the fluctuations to reveal the
underlying wave form.
The most common technique available is "smoothing". These techniques
serve to surpress the noise and to extract real trends and patterns.
Note that smoothing and averaging are not the same:
- Averaging ends up with fewer actually data points
- Smoothing preserves the number of data points but lowers
the amplitude of the noise by coupling the data points.
Techniques: